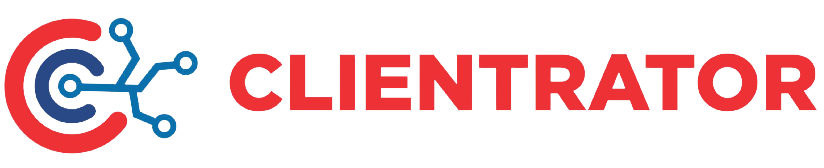
Clientrator is a comprehensive AI & ML driven data analytics tool from G-Square which solves multiple use cases of predictive analytics.
Clientrator has been made to meet your expectations
No code-Low code approach
Clientrator does not require any coding expertise, machine learning models and analysis can be done using a no-code approach.
USP
Model Building & Model Output: discovers patterns within the training data, and it outputs an ML model which captures these patterns and makes predictions on new data.
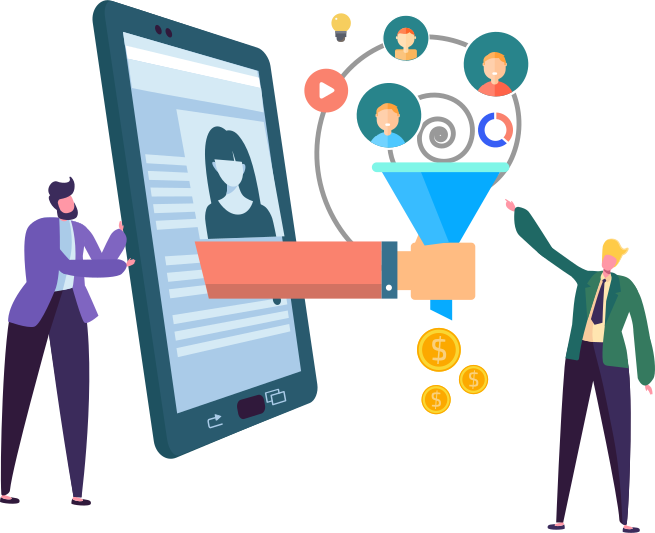
USE CASE & BENEFITS
Cross-sell & Up-sell
It helps you to determine the group of customers who can be targeted for cross-sell and up-sell based on their past history and purchasing patterns.
Retention Analytics
The process of analyzing user metrics to understand how and why customers churn. Retention analysis is key to gain insights on how to maintain a profitable customer base by improving retention and new user acquisition rates.
Customer Profitability Analytics
It allows businesses and lenders to determine the profitability of each customer or segments of customers, by attributing profits and costs to each customer separately.
Lead Analytics
Acts as a report card for your sales and marketing efforts, by tracking lead performance
Credit Risk Scorecards
A credit scorecard is a model used to estimate how likely a credit applicant is to repay a loan based on information about the applicant’s credit history.
Market Basket Analysis
Data mining technique used to increase sales by better understanding customer purchasing patterns
CLIENTRATOR FEATURES
Exploratory Data Analysis (EDA) : is an approach to analyze the data using visual techniques.
Model Building & Model Output: discovers patterns within the training data, and it outputs an ML model which captures these patterns and makes predictions on new data.
The predictive output can be tracked within the tool as an output or it can be integrated with external campaign tools or CRMs or any other systems to track model progress. The model keeps on learning on an ongoing basis.
Time to give it a try
Time to give it a try
As we told you, there’s no substitute for hands-on experience. Try Clientratorfor free
Request a Demo Try it on your own